In this post, we will talk about easy ways to draw binary trees.
Binary Trees with Graphviz
This first method is to use the open source Graph Virtualization Software - Graphviz.
Let’s start an example of drawing a binary tree as shown in the following figure.
First of all, create a file and then type in the following content, and save it as bt.dot.
graph{
a -- b;
a -- c;
c -- d;
d -- e;
}
Then, open a terminal and use cd command to come to the directory where bt.dot locates, then type in the following command in the terminal, and run it.
dot -Tpng bt.dot -o bt.png
After running the command, you will find bt.png in the same directory as bt.dot. This figure is shown below. (Note that, it seems that Graphviz on Mac does not support png yet, so if you use Mac to go through this example, we can change png to ps or any other types that are supported.)
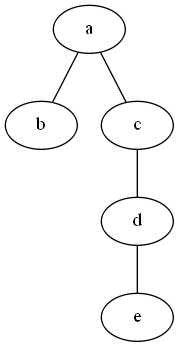
Of course, Graphviz is much more powerful than that, if you are interested you can refer to the following tutorials:
However, from the above simple example, you might already notice that, Graphviz treats left child and right child indistinguishably. So if you want to distinguish between them in your case, it would not be a very good solution. There is no doubt that Graphviz provides you the power to customize the layout or even location of each vertex, however, it would be inconvenient if you have to specify the location for each vertex. And I feel surprised that Graphviz does not provide any easy ways to achieve this goal. On Stackoverflow, someone provides a solution to achieve this goal (I have not tested the solution, I am not sure whether it works or not), but the solution is not perfect.
Binary Tree with Tikz
Tikz-qtree provides a simple solution to draw binary tree in which left and right children are distinguishable.
Let’s draw the same binary search as in the above example.
First of all create a file and type in the following content and then save it as bt.tex (note that, the original Latex codes are copied from Stackexcahnge)
\documentclass{article}
\usepackage{tikz-qtree}
\begin{document}
\tikzset{every tree node/.style={minimum width=2em,draw,circle},
blank/.style={draw=none},
edge from parent/.style=
{draw,edge from parent path={(\tikzparentnode) -- (\tikzchildnode)}},
level distance=1.5cm}
\begin{tikzpicture}
\Tree
[.a
[.b ]
[.c
\edge[blank]; \node[blank]{};
\edge[]; [.d
\edge[]; {e}
\edge[blank]; \node[blank]{};
]
]
]
\end{tikzpicture}
\end{document}
Then, compile bt.tex. The following shows what the binary tree looks like.
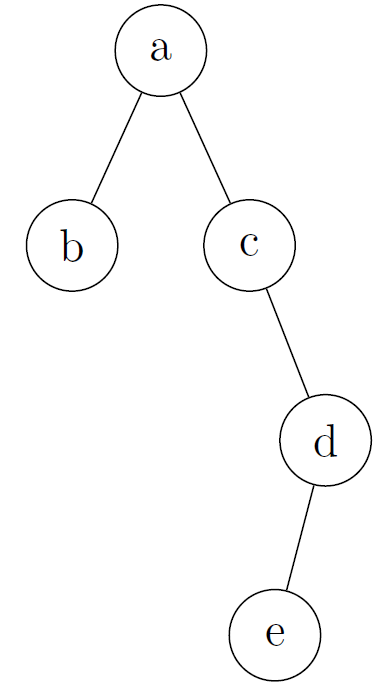
The following give some tutorials on how to draw beautiful figures with Tikz: